Action Execution
To achieve our goal of enabling a robotic agent to handle unkown task variations by parameterising general action plans using web knowledge, we employ the following architecture:
In general, the robot needs to have access to a general action designator of cutting that can be parameterised. When the robot is given a task request, it can either query the graph database with the knowledge graph directly via its SPARQL REST API or use a knowledge framework with additional functionalities such as the KnowRob knowledge processing system1 and pose Prolog queries, which then are translated to SPARQL queries. More information on the different ways of querying the knowledge graph can be found here.
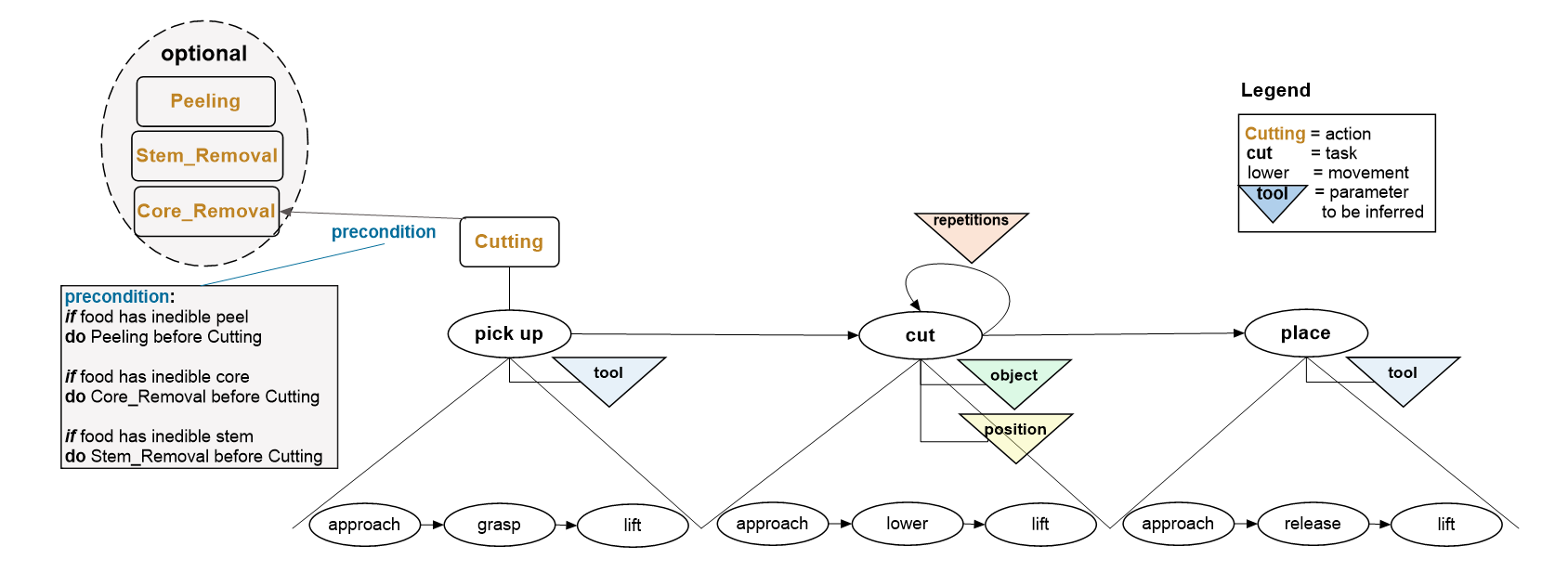
We use PyCRAM as the robot’s cognitive architecture. It is the Python3 implementation of the CRAM cognitive architecture2 and expresses its programs as symbolic plans (so called designators) outlining the robot’s behaviour. During execution, a designator resolver is used to refine the generalised instructions into specific instructions the robot can follow. One way of resolving a designator includes querying the knowledge graph to gather concrete values for abstract parameters based on the current environment, object composition and task to execute.
References
M. Tenorth and M. Beetz, ‘KNOWROB - Knowledge Processing for Autonomous Personal Robots’, in 2009 IEEE/RSJ International Conference on Intelligent Robots and Systems, St. Louis, MO, USA, Oct. 2009, pp. 4261–4266. doi: 10.1109/IROS.2009.5354602. ↩︎
M. Beetz, L. Mösenlechner, and M. Tenorth, ‘CRAM - A Cognitive Robot Abstract Machine for Everyday Manipulation in Human Environments’, in Proceedings of the 2nd IEEE/RSJ International Conference on Intelligent Robots and Systems (IROS 2010), R. C. Luo and H. Asama, Eds., Taipei, Taiwan: IEEE, 2010, pp. 1012–1017. doi: 10.1109/IROS.2010.5650146. ↩︎